The design and creation of a new robotic device for phenotyping plants is a double-sided boon to agriculture. On one hand, it is indicative of the enormous potential of the autonomous systems now in development for farm applications. On the other, it’s a huge leap forward for researchers and plant breeders and their ability to develop corn hybrids (and other crop varieties) with better tolerance to different environmental stresses.
Dr. Gui DeSouza, an associate professor of electrical engineering and computer science at the University of Missouri at Columbia, is part of a multidisciplinary team that has been working together for five years to perfect this technology. DeSouza and students — particularly Ali Shafiekhani — from his Vision-Guided and Intelligent Robotics (ViGIR) Laboratory have worked with several others to develop this system, in co-operation with the National Science Foundation.
Read Also
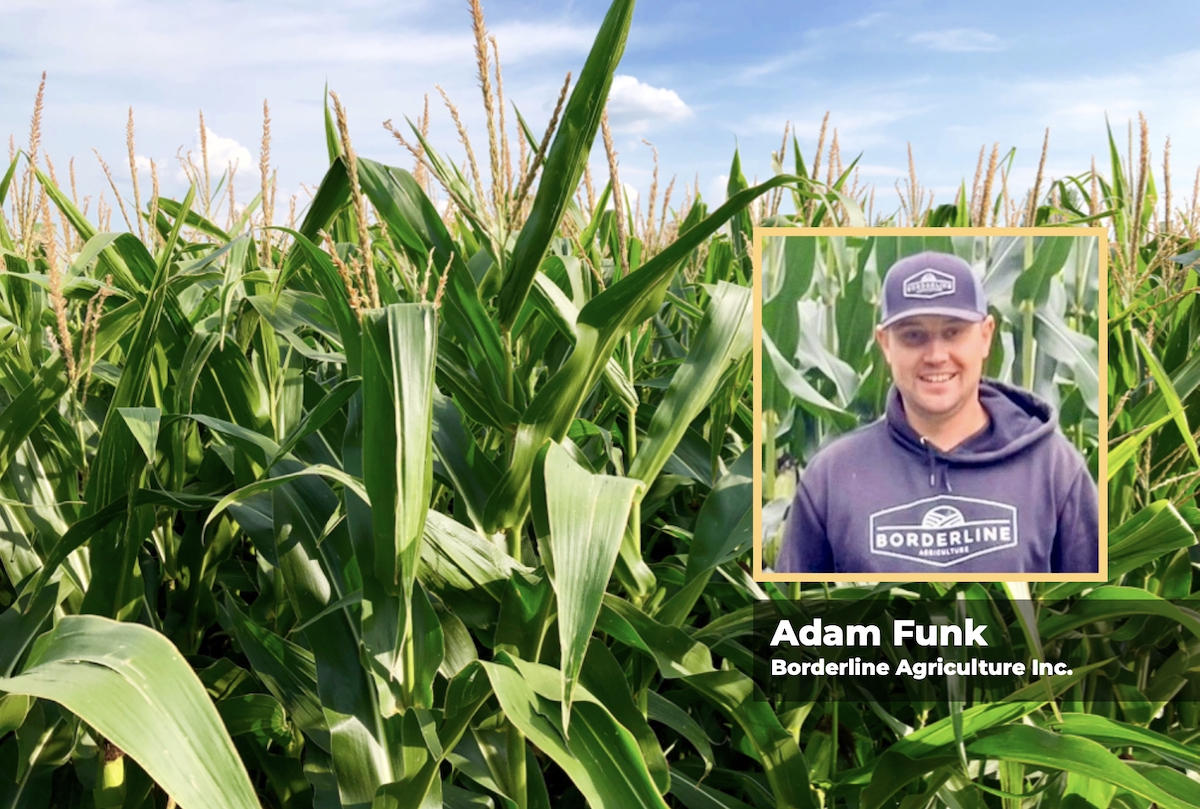
Agronomists share tips for evaluating new crop products and tech: Pt. 3
With new products, new production practices and new technology converging on the agriculture industry at a frenetic pace in recent…
Work began back in 2013 on a robotic unit that originated with a grant proposal tied to another application. In March 2014, the university was awarded a multi-million dollar grant to study climate change and corn’s ability to grow under drought conditions.
By 2015, DeSouza and his team, which included Felix Fritchi and other researchers from the College of Agriculture, Food and Natural Resources and the Danforth Plant Science Centre in nearby Creve Coeur, were working on a small robotic unit that could move easily and quickly through a field. That work was a progression from similar imaging and collecting done in a greenhouse setting. The next year, they added a tower unit to the system, with a mobile robot design that was a little bigger, carrying a camera that could record three-dimensional (3D) images of plants.
The 2017 growing season is the second year for the tower and the third for the mobile robot unit, with the 2018 and 2019 growing seasons still part of this particular project.
They are breaking new ground. There is no commercial technology in use anywhere for this particular purpose.
The tower is capable of detecting portions of a field down to a 60-foot radius where stress could be affecting growth, including drought or heat stress. Then it guides the robotic unit into that section of the field to take photos and collect specific measurements at three different heights before returning that data to the laboratory for the development of 3D models of the plant. Temperature, humidity and gas exchange rates are just three of the properties detected by the current design.

“Both the tower and the mobile robot collect 3D data of the field, so we collect 3D models and create 3D models of the plant,” says DeSouza, noting the extraordinary capability for capturing images from different angles under different conditions. “In the field, you have wind or other changes. You take one snapshot from one viewing angle, and take the next one for the next viewing angle, and the plants can change shape. So creating 3D models from deformable objects like that is a very big challenge in computer science.”
Considering the capability of collecting different data sets from different heights, and its ability to take thousands of phenotypic measurements from across a field, it’s easy to grasp the enormous potential this technology holds. Three-dimensional modelling of this sort can help provide researchers and plant breeders with volumes of invaluable field-based data, to be used in the development of various stress-tolerant hybrids or other crop varieties. DeSouza’s research has concentrated on the robotic unit’s applicability in corn and soybean, but many other crops could be candidates for such in-depth modelling.
Sky’s the limit
What really sets this technology apart is its adaptability. Multiple towers can be brought to a field to cast an even wider perspective on a crop’s performance. It also allows for greater efficiency in taking these measurements, and unlike unmanned aerial vehicles (UAVs) or drones, this robotic unit can take measurements any time — day or night.
“Field phenotyping used to be done — and is still done — by humans, but they have to go into the field to collect data by hand and it’s a lot of stress,” says DeSouza. “They can’t collect the amount of data that we need to make the conclusions and to address the questions that we’re trying to address. It’s a lot better to train people to analyze the data, and understand what the robots are collecting, than to just collect the actual data.”
It’s not that DeSouza is trying to replace workers or create a level of social uneasiness. Instead, he believes they’re creating the system that eases the workload for people while increasing the efficiency of testing conducted on plants.
That’s the goal — to employ the robots that can collect the data, in a quantity and quality that has never been achieved before, especially in the field. There’s been a lot of work done in the greenhouse, where it’s easier to deploy or install equipment, and where something like 3D modelling is much simpler, because there are fewer environmental variables that can have an impact on the measurements. But in the field, the challenges are much larger and more variable.
In the field, they can also collect data from 3D images, including physical traits such as leaf angle, leaf area, and distance between leaves, because those are distinct, important factors in corn. DeSouza is also looking to do similar work in soybeans, where they can measure pod size, seeds per pod and number of pods on the plant. It’s conceivable that, as with UAVs, different traits or properties could be detected, simply by changing filters on the cameras, adding even more potential to this technology. Nutrient uptake, weed intensity, insect thresholds or the progress of diseases — all have the potential to affect a crop’s development. And all could be incorporated into data collection at varying times of a growing season.
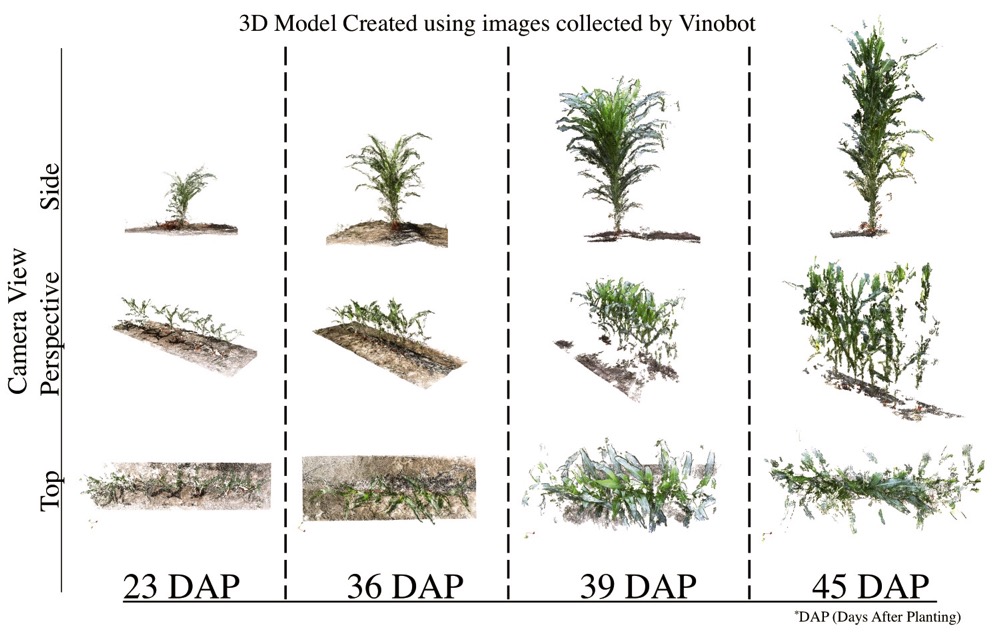
Initially, this information is being looked to as a valuable resource for researchers and plant breeders, but it could eventually be of use to in-field personnel too, including agronomists and farmers. Unlike soil testing, the frequency or area of sampling isn’t an impediment. In fact, as the frequency of sampling and imaging increases, the cost per acre shrinks while the data collected are far more comprehensive in their scope and detail.
“Also in the past, doing field phenotyping was usually a destructive process, because the person had to grab the corn plant, take it out of the soil and bring it back to a place where you could do the measurements,” says DeSouza. “You had to have a lot more plants to be able to afford every measurement. But now, we don’t have to do that. We can send out the robot at a certain date of the plant’s growth, and then again a few weeks later, or at the end of the season.”
They actually came up with the idea for the tower because the use of UAV approach was becoming more complicated with the need for certified flight plans filed ahead of time. Curiously, the primary goal for this design — not surprisingly given that DeSouza works in the department of electrical engineering and computer science — was to achieve autonomous movement. As a result, they can collect more observations and then correlate them with the biology of the plants.
“There’s no end to how we can adapt the current system and evolve it,” DeSouza says. “We want faster robots, we want robots that can do specific tasks, more autonomously, more efficiently, and go to the plant site to do the same thing. Once we address those questions about how the plant behaves in the field relative to the greenhouse, then we want to study the genotype of the plant. We want to study how we can breed different plants that will respond to those stresses that we observed before, and create new types of plants that can tolerate different stresses.”
The climate is changing, adds DeSouza, and there are more extremes of weather, be it drought stress or excessive moisture. By all indications, the Corn Belt in the U.S. Midwest is migrating farther north, while parts of the Canadian Prairies are becoming more conducive to growing corn and soybeans. The summer and day cycles remain the same but that doesn’t mean corn production can simply shift to those northerly regions: growers and the industry must still learn to deal with the conditions as they arise.
“We are always trying to get better and improve, and again, the population is growing, we have to feed more people and the demands are pushing us further,” says DeSouza. “Even during the process, it’s not that we’ll wait until 2019 to disclose the results — there are other groups that we work with. We report to the NSF constantly, with how people can access the data and how it’s formatted, how they can interpret the data and how they can download them.”
For more content related to drought management visit The Dry Times, where you can find a collection of stories from our family of publications as well as links to external resources to support your decisions through these difficult times.